Dominik Seisser is a senior AI practitioner and expert for Natural Language Understanding (NLU). In his recent interview with the Turning Magazine, he speaks about the relationship between AI research and application, his challenges as an AI lead, and explains how Cognigy keeps up with Big Tech.
Dominik, what does a Head of AI do?
As the Head of AI at Cognigy, I lead a team of machine learning experts, AI developers, and computer linguists. My team is responsible for our company’s self-developed NLU engine that is at the heart of our offering, Cognigy.AI. Part of my job is to also stay up-to-date on the latest technology advancements in AI and leverage leading-edge research for our application.
What exactly does Cognigy develop?
We make a commercial platform for customer service automation designed for large enterprise organizations. Our clients use the software to build smart chat and phone bots to provide real-time support for human agents in contact centers or to offer instant, intelligent help to consumers via messenger apps. Natural language processing is the centerpiece of our stack: It enables the software to understand users’ expressions in natural language and communicate back to users accordingly. In a nutshell, my team makes machines understand humans. Or more precisely, we teach the machine to understand what we want it to understand.
Do you have separate teams for voice and text-based bots?
At present, spoken language is pre-processed by speech-to-text engines so that the NLU receives a text input just like it would in a text-based channel. This has several advantages that outweigh the benefit of going end-to-end on voice. Even the business logic of a bot can be shared across channels. What is different is the conversation design of text and voice bots —spoken replies need to be shorter and more precise. Visuals, buttons, or links are unavailable. And users cannot simply scroll back up, so a voice bot needs to provide clear guidance to end-users.
Take a sneak peek of Cognigy.AI's voice and text-based bots in action.
What is the relationship between AI research and AI application in business?
Academics built the foundation on which we designed our application. Without AI research, there would be no Cognigy NLU. But it is also important to note that research is mostly focused on the fundamentals of AI. Building applications with AI opens a vast and excitingly unexplored field of questions.
Interestingly, there is surprisingly little research in regard to real-world AI applications. One reason is that it is not easy to access real usage data and make that subject of research. That is certainly one of the advantages we have in our R&D efforts. The feedback loop between building something and observing it in action is really short. We are releasing a new NLU version about every four weeks, so we can build, measure, and improve with a much faster turnaround time than a large-scale research project.
Major players like IBM, Google, and Microsoft invest billions of dollars into AI. How can Cognigy keep up?
Indeed, we are a bit in a David vs. Goliath situation: We are small compared to Big Tech vendors, but we can be faster, leaner, and extremely focused on our specific use cases for applied AI. That allows us to rapidly adopt the latest technology.
Some aspects of AI such as the training of massive language models with supercomputers or building STT/TTS engines require extremely large investments. But that is not our focus. In many regards, we and our customers benefit from services and advancements that would be impossible without the Silicon Valley billions. After all, it is more of a co-existence of smart services and research results than a direct competition of technology. That enables us to concentrate our R&D efforts precisely on building the best possible product for our clients’ use cases.
It is like the market for super sports cars. Sure, Ferrari cannot beat Toyota in volume or revenue. And the Ferrari portfolio is less versatile than Toyota’s. But it’s still possible to build a highly competitive car with a small, dedicated team in a certain market. On the racetrack, a professional driver prefers the F8 over the Corolla.
See how Cognigy competes with the different NLU engines.
If you promise top performance, you need to prove it. Can you measure how good your NLU is?
We can, and we constantly do that based on more than 500 million human/machine interactions per year handled on our platform. So we have a lot – fully anonymized – data about successfully or unsuccessfully completed transactions. We know where friction occurs in a conversation or which user expressions are less understood by the machine. That data feeds back into the development of our NLU, allowing us to continuously measure and improve performance, based on real-world input.
We even go one step further and provide our clients with a prediction of NLU performance. Each of our customer runs their own NLU instance, specialized for their specific use case and based on individual training data. Our software analyzes how precise a model is and where additional training will have the most impact. And it can even predict on a granular level if e.g. adding a specific training sentence will improve the NLU’s accuracy or not. All of that requires a lot of complex data science - but for the business users, this analysis is visualized with a simple traffic-light indicator. That is a good example of a Cognigy feature that you will not find with any large vendor.
How do you evaluate your solution in comparison to other NLU vendors?
My team regularly runs benchmark tests to evaluate how our solution compares to others. As we— and most other vendors— constantly tweak and extend our technology, it is critical to have a standardized, highly-automated testing process in place.
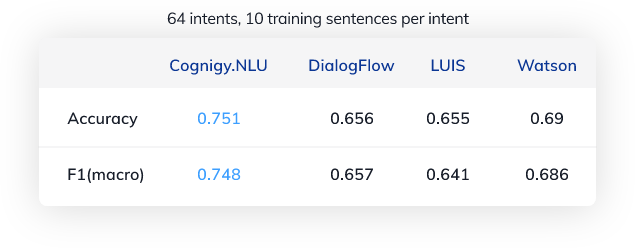
What is even more important is to use a large, unbiased dataset to get reliable results. It would be easy to select test datasets in a way that the own NLU outperforms any other by orders of magnitude. However, such whitewashing would sabotage our efforts to remain in an objectively and verifiably leading position. Therefore, we value a number of independent test data from academic institutions and research groups which are fully independent of us or any other vendor. My team is proud that we can rightfully claim a leadership position in regard to the accuracy and reliability of our NLU among leading commercial vendors. One of our particular strengths is few-shot learning, which helps our clients achieve high recognition accuracy with limited training efforts. It is also important to be transparent about the test data and process. That is why we provide test details open-source on Github, so our findings can be replicated by anyone.
What are your biggest challenges – technically and as an AI team lead?
A constant challenge is scaling the application to the requirements of the real world. It is one thing to build a demo or a slick proof-of-concept for an AI product. But as soon as a tool is in production, everything becomes a lot more complex: Hundreds of concurrent business users training the NLU. Batch uploads with millions of entities that the NLU needs to learn. Steep and unexpected spikes in end-user requests. And the underlying technology needs to deliver top performance, in global deployments, with minimum latency and guaranteed up-time. On top: Being a component of Cognigy.AI, the NLU is part of a much larger stack. It is entangled with front- and backend, there are countless dependencies that would not occur in a lab situation. All of this requires a lot of solid engineering.
Speaking of me as an AI team lead, finding the right talent is currently one of my toughest challenges. There is a lot of enthusiasm and excitement for AI, but it is challenging to learn and master AI application design to a level that is needed for a senior position in a fast-paced company like Cognigy. Hiring a jack of all trades, a top AI-scientist with a track record of AI application development is close to impossible.
What do you recommend to students aiming for a career in AI?
To me, a real passion for AI is the best foundation for an AI career. It is absolutely fascinating to see how these man-made machines work, what they are capable of and what their potential is. My advice: Follow your passion to understand the technology in-depth and how you can leverage the power it may yield. Build things. Combine the learner’s spirit of “starting from scratch” with a good dose of practical accomplishment and result orientation.
An advanced degree in a technical field certainly helps to get your foot in the door. At a minimum, sit in a few seminars with top researchers in the field and witness what human minds can do. All of this helps to appreciate how little you understand and how deep the rabbit hole of AI research really is. My take: Combine academic and theoretical approaches with hands-on building and prototyping to experience, learn and understand what AI can do. And be confident that there has never been a greater time for a career in AI.