The 7 action areas for auditing according to AIC4
As of May 2021, the AIC4 criteria catalogue comprises seven different action areas that cover the minimum requirements for auditing AI applications. They are supplemented by the requirements of the Cloud Computing Compliance Criteria Catalogue (C5) already described in the preceding chapter.
In the following, we provide an overview of the significance and goals of the seven action areas. For a detailed look at the implications of each action area for customer service automation, please refer to our free whitepaper. There, we have also included use cases from Cognigy's practical experience to give you an understanding of concrete measures for meeting the respective AIC4 action area.
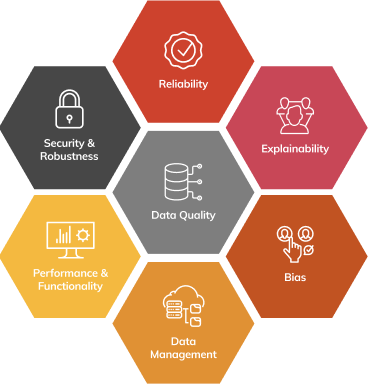
Action area 1: Security and Robustness
This action area deals with protecting AI applications from manipulation attempts. The ability to react to continuously changing systems and environmental conditions plays a decisive role here. In order to detect malicious attacks, suitable tests must be developed and regularly performed on the part of the AI solution provider. Furthermore, the focus needs to be put on proactively implementing measures against targeted attacks. Through tests and measures, the AI application should gain robustness, the processed data should be protected, and the ongoing secure development of the algorithms utilizing training data should be ensured.
In the area of Conversational AI, meeting the criteria of this action area will lead to increased integrity of virtual agents (phone and chatbots), protection of personal data, reduction of manipulation risks, and secure operation of AI applications in the cloud.
Action area 2: Functionality and Performance
The objective here is to define and measure the performance of an AI service in its particular area of application. This is done by relying on appropriate procedures for training algorithms as well as for validating and testing AI applications. The metrics proposed in the AIC4 catalogue for, inter alia, the accuracy, sensitivity, and the error rate of the AI service, are used, on the one hand, to check the functionality and performance scope agreed upon with contractual partners and, on the other hand, act as a basis for future performance improvements.
In the area of Conversational AI, meeting the criteria of this action area will lead to an increase in reliability of virtual agents in customer use (e.g., during peak load), increased quality and performance of phone and chatbots after each training iteration, and the use of the most advanced and plausible machine learning models.
Action area 3: Reliability
This action area is intended to ensure the reliable operation of the AI service in production environments. In addition, processes must be established to investigate errors and failures of the service. This includes, for example, the provision of adequate resources for maintaining the AI, logging procedures, backups, and processes for error handling. In this context, partial use is made of the C5 catalogue criteria.
In the area of Conversational AI, meeting the criteria of this action item will lead to better accessibility of virtual agents (24/7), continuous maintenance of Conversational AI platforms, and close monitoring of AI services.
Action area 4: Data Quality
The major issue is that data used for ML model development, intent training, and AI service operation must meet certain quality standards. For example, training data should be of appropriate quality and quantity to adequately train the AI for the corresponding area of application. In addition, training data must be easily accessible and consistent in structure. This has a significant impact on the explainability of AI models ("explainable AI").
Meeting the criteria of this action area will lead to better virtual agents in the area of Conversational AI, as the quality of the training data is crucial for the quality of the phone and chatbots. In addition, processes for data acquisition, qualification and evaluation are followed and possible biases (so-called "AI bias") in the ML models are avoided.
Action area 5: Data Management
This control area establishes the framework for the structured collection of data and the use of data from trusted sources. The AI service must also establish a framework for developing and operating its service, using training data. This includes, for example, adequately protecting the training data from access through unauthorized individuals. The training data must also be used for its intended purpose and must be documented.
In the area of Conversational AI, meeting the criteria of this action area will lead to better documentation and more transparency in the training data, legal protection, as only data that also meets legal requirements and is approved may be used, and the establishment of granular access rights in the AI system.
Action area 6: Explainability
The decisions made by algorithms in the context of an AI service must be comprehensible. If necessary, experts must be consulted and suitable techniques must be used for the assessment. Among other things, it must be possible to explain the purpose and operation of the machine learning model in use. This information must be documented and prepared in such a way that an evaluation by external parties, for example, technical and industry experts, but also users, is possible. Only when these actions take place an AI becomes "Explainable AI".
In the area of Conversational AI, meeting the criteria of this action field leads to a better traceability of the decisions of virtual agents. It also creates an opportunity to have ML models externally verified. This accelerates the use of conversational AI technologies because reservations in the company are reduced more quickly.
Action area 7: Bias
In this action area, the possible bias of the AI service is to be identified. This involves, for example, direct and indirect bias as well as systemic and statistical bias. The AIC4 is not explicitly designed to assess biases in the code or training data from an ethical perspective. Rather, the aim is to uncover possible vulnerabilities by applying mathematical methods (for example "adversarial debiasing," "prejudice remover," etc.) and thus make them transparent to the user of the AI service. An assessment must then be made by the user. As part of the AIC4 audit, the AI service operator is also required to reduce any AI bias identified using specific algorithms.
In the area of Conversational AI, meeting the criteria of this action field leads to a reduction or elimination of bias (e.g., discrimination), equal treatment of users and intents - regardless of origin or dialect, and factual-rational communication between humans and virtual agents.
"The AIC4 is based on the BSI's globally recognized Cloud Computing Compliance Criteria Catalogue (C5). This integration makes it possible to essentially focus on the AI-specific aspects in audits according to the AIC4, and to refer to existing processes and controls from the C5 environment at important points, for example, in the operation of the AI solution."
Hendrik Reese, Director, Artificial Intelligence - PwC Germany